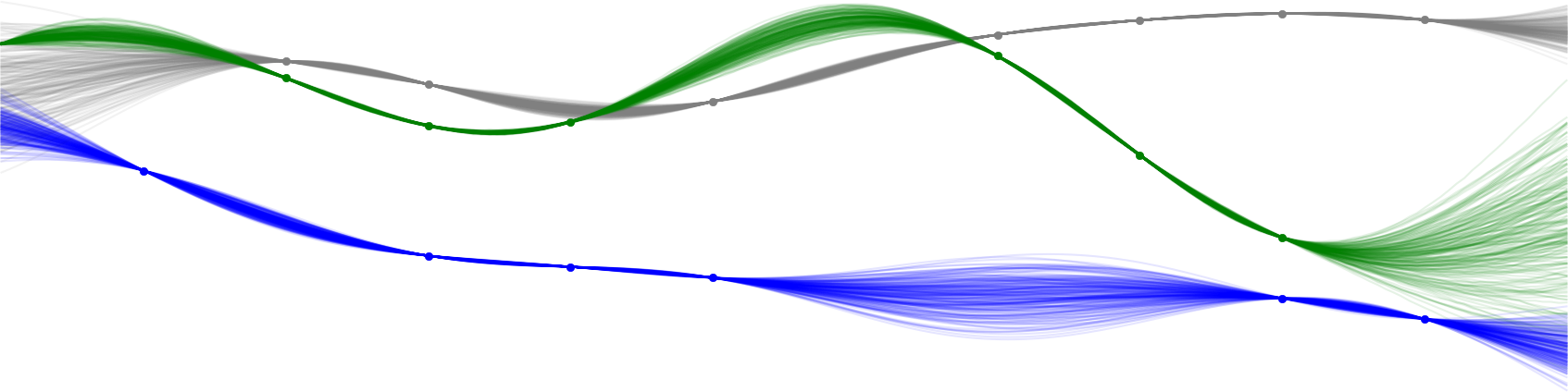
Recent Publications

The RESP AI model accelerates the identification of tight-binding antibodies
Nature Communications, February 2023
In this paper, we use a combination of high-throughput, high-quality data generation and uncertainty-aware modeling to perform in silico directed evolution — and design the tightest binder to a key cancer target described in the literature.
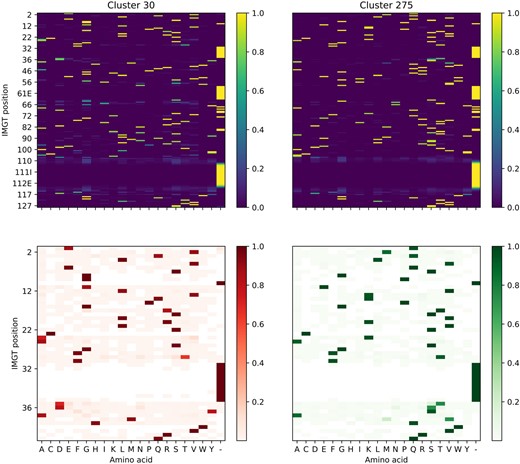
Fast, fully-interpretable, state-of-the-art human-likeness scoring for antibodies for reduction of immunogenicity risk
Bioinformatics, May 2024
In this paper, we first accelerate antibody numbering by nearly two orders of magnitude compared with existing tools. We next introduce a fully interpretable model which achieves state-of-the-art performance for distinguishing human antibodies from nonhuman and explore how our model can be used to improve developability in early-stage discovery.
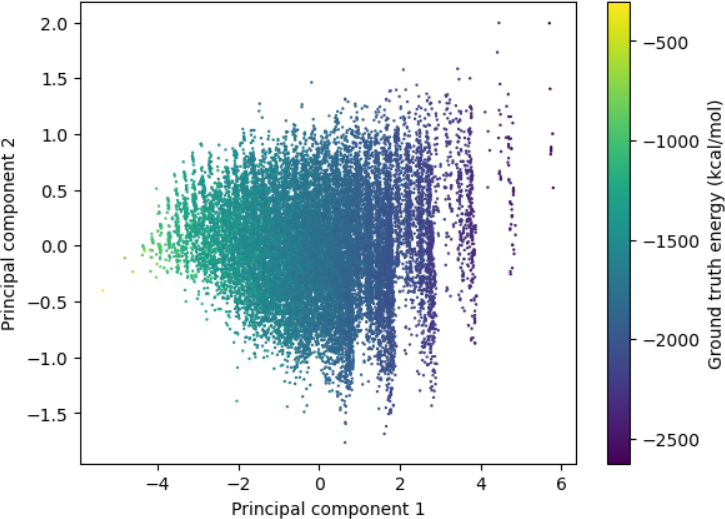
Scalable Gaussian process regression enables accurate prediction of protein and small molecule properties with uncertainty quantitation
Journal of Chemical Information and Modeling, July 2023
In this paper, we illustrate how better algorithm design can take an architecture traditionally regarded as non-scalable — a Gaussian process — and convert it into a model that can compete with deep learning on large datasets. We also explore how uncertainty-aware Gaussian processes can be combined with traditional AI and demonstrate how an uncertainty-aware model can be used to “design” a protein with a desired property in an automated way, without any human intervention.