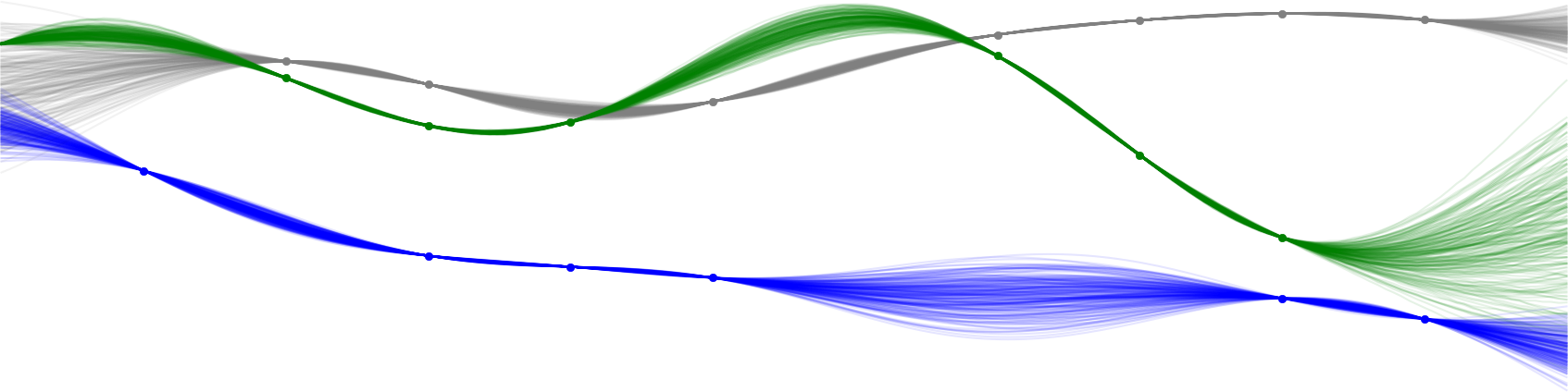
Finding the right molecules is hard.
Drug discovery has traditionally relied on expensive repeated rounds of trial and error: keep tweaking until you find something that works. This approach might be sufficient, if tight binding to a specific target were the only desired property, but it’s not — you need solubility, stability, pharmacokinetics, tissue penetration and bioavailability for small molecules, low propensity for immunogenicity for antibodies, and other properties as well. That’s one reason why hit-to-lead optimization often turns into a long-drawn out process.
Current machine learning techniques have helped but aren’t likely to solve this problem alone. Traditional AI models were developed to deal with problems where data was high-quality, abundant and cheap, unlike drug discovery where data is typically expensive, low quality and scarce. Black-box AI models trained on these kinds of datasets frequently make predictions that are both wildly overconfident and wrong — and since the company developing the AI model doesn’t completely understand how it works, they may or may not be aware there’s a problem. Most companies hoping to apply AI to drug discovery thus end up substituting one kind of guesswork for another.
We can help.
At MAP Bioscience, we’ve developed a unique toolkit for tackling the challenge of drug discovery.
- AI that knows what it doesn’t know. Unlike most companies, we use uncertainty-aware AI that is aware of its own limitations. We never have to guess, because we know whether a prediction is reliable or not as soon as it’s generated.
- High-quality, high-throughput data generation. We have extensive experience designing experiments to generate high-quality data for new targets in high-throughput, enabling us to quickly acquire the data we need to model a new problem.
- Accurate, uncertainty-aware antibody developability assessment and prediction. We’ve mined data for over a billion antibody sequences to yield predictive models that can quickly determine whether a sequence has what it takes to make a clinical candidate.
- Fast, reliable simulations. Most simulations of how molecules behave in solution rely on crude approximations of the underlying physics. We’re building software that can run highly accurate simulations at speeds and scales beyond any currently possible. This enables us to perform some key experiments on a computer rather than in the lab.